Decoding Student Success: Insights from Blended Learning in International Studies
As the International Studies program at Leiden University continues to evolve, it faces new challenges in meeting the diverse needs of its growing student body. With an increasing intake of students and a wider range of learning styles to accommodate, understanding the varied learning behaviors of first-year students has become crucial. Recognizing that each student’s approach to learning is unique, a team of data scientists, researchers, and educators embarked on an ambitious project to analyze student engagement patterns.
The study focused on two compulsory courses: Academic Reading and Writing (ARW) and Sociolinguistics (SL). These foundational courses offered students a mix of online and offline learning experiences. The research wasn’t just a casual look into how students navigated these courses; it was a deep dive into their behaviors, using data-driven techniques. Researchers wanted to know: What are students really doing when they learn, both online and offline? And how can this information help us support them better?
The Quest to Understand Learning Behavior
With 472 students initially enrolled for both courses, and 469 consenting to participate, the project quickly got off the ground. The researchers were thorough in their data collection, using a variety of sources. From learning management systems like Brightspace to online platforms like Kaltura and Webcast, they captured detailed online engagement data. To supplement this, they gathered offline activities through bi-weekly logbooks and interviews. The team also performed an array of statistical analyses, employing advanced techniques such as Generalized Additive Models (GAM), XGBoost for prediction, and clustering methods to find patterns in student behavior.
What emerged from this intensive study were three distinct clusters of student engagement:
- Cluster 1 (52%): Less active students, highest course dropout rates.
- Cluster 2 (28%): Most active students, achieving the highest grades and the lowest dropout rates.
- Cluster 3 (20%): Moderately active students, but with particularly high engagement in video content.
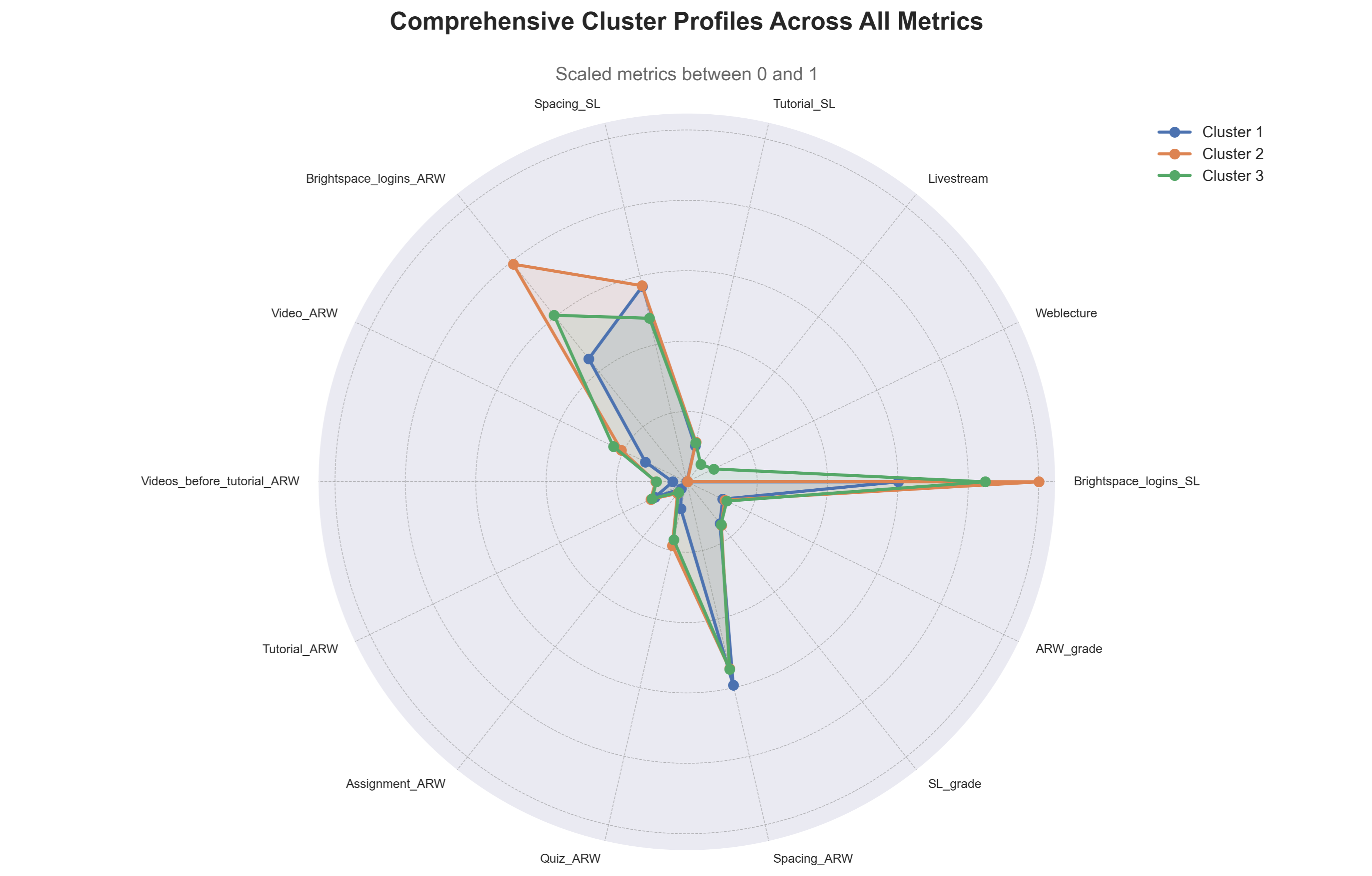
Comparative visualization of student engagement profiles across three clusters, displaying normalized metrics. Image courtesy of Arian Kiandoost
Predicting Success
The researchers weren’t just content with identifying clusters. They wanted to predict what behaviors led to academic success. Using machine learning models like XGBoost, they analyzed key factors that contributed to higher grades.
In Sociolinguistics, variables like the number of tutorials attended, average quiz scores, and assignment grades were strong predictors. For Academic Reading and Writing, tutorial attendance, video engagement, and completed assignments emerged as important drivers of student success. However, these factors explained only a portion of the grade variance—35% for SL and 30% for ARW—indicating the complexity of predicting academic performance.
The Challenge of Offline Learning
Although the study achieved valuable insights into online behaviors, capturing offline activities presented significant challenges. Despite incentivizing participation, only a small fraction of students consistently completed the bi-weekly logbooks. Those who did participate offered a glimpse into offline learning. For example, no significant correlation between online and offline engagement could be established based on collected data. This finding deserves further discussion and future work, as it may imply divergent study strategies: students who engage a lot online do not necessarily engage a lot offline as well.
Overall, however, a dominant study strategy among students was to take and review notes. These notes were the single most important resource for exam preparation. Surprisingly, some students reported they could succeed without attending the lectures or reading the literature, relying more heavily on digital resources like lecture slides and webcasts.
Beyond the Data: The Human Element
The project also revealed personal factors that influenced learning, some of which were harder to quantify. Mental health challenges were a significant barrier for a substantial minority of students, while time management issues added to the stress. Despite these obstacles, the majority of students were motivated and keen to succeed in the program, often refining their learning strategies as they progressed through the semester.
One important observation was that formative feedback played a crucial role in helping students improve. Students who used feedback from midterm assessments tended to engage in deeper learning and self-reflection, setting themselves up for better long-term success
Implications for the Future
So what do these findings mean for educators? The study offers several actionable insights. One major recommendation is to develop a data-driven early warning system to identify at-risk students—particularly those in Cluster 1—early in the semester. By flagging students who are falling behind in engagement or academic performance, instructors can intervene with targeted support.
Additionally, the results point to the need for flexible course structures. Students in Cluster 3, for instance, engaged more heavily with online content but performed similarly to the highly active students in Cluster 2. This suggests that a one-size-fits-all approach to course design might not be the best way forward. Instead, offering multiple pathways to success—whether through videos, readings, or peer collaboration—could cater to different learning styles.
A crucial implication from the results is the importance of intermediate assessments and personal feedback. The quizzes in Sociolinguistics proved to be a powerful way to foster learning and motivate students. Such assessments, whether used in-class or as part of the online component, are efficient ways to provide personalized feedback and encourage ongoing engagement with the course material. Implementing similar strategies across other courses could significantly enhance student learning and performance.
Furthermore, the study advocates for a more integrated student support system, connecting academic performance with mental health resources and academic advising. By offering a holistic support network, universities can help students overcome both academic and personal challenges, leading to improved overall outcomes.
Lastly, incorporating formative quizzes and interactive components into both online and offline learning would encourage deeper understanding and participation. This approach not only reinforces key concepts but also provides students with immediate feedback on their progress, allowing them to adjust their learning strategies as needed.
Moving Forward
While the study has laid a solid foundation, the team is already looking ahead. Future plans include developing a predictive model that can flag at-risk students before problems arise, testing the model’s accuracy using future student data, and designing intervention strategies that can be deployed when needed. Additionally, a longitudinal study is proposed to track student progress over several years, providing deeper insights into the long-term effectiveness of these interventions.
In conclusion, this project has illuminated the nuanced ways in which students engage with their studies. Although the clusters of learning behavior were not sharply distinct, they still offer valuable guidance for improving course design and student support. By leveraging these insights, Leiden University and other institutions can create more responsive, data-informed educational environments that meet the diverse needs of their students. The journey towards fully understanding and optimizing student learning has only just begun, but this project has certainly contributed a meaningful step forward.