Publications
Using data to analyse WFP’s digital cash programme in Lebanon
Increasingly, the World Food Programme’s assistance is cash-based. The large quantities of data generated by transferring cash digitally could help transform humanitarian programme design, implementation and monitoring.
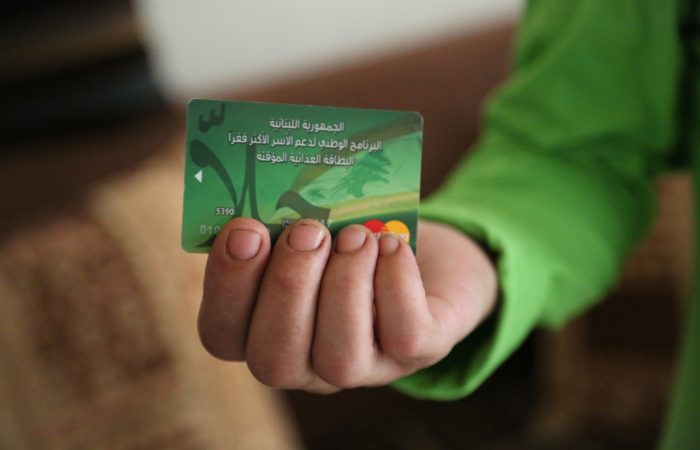
Humanitarian programmes have recently started to shift from delivering in-kind aid – such as food, clothes and tents – to cash-based assistance. According to the New York Times, before 2015, 99% of the world’s humanitarian assistance came in the form of goods. By last year, that number had decreased to 94%.
As of 2016, just over a quarter of the United Nations World Food Programme’s (WFP) assistance worldwide is cash-based (WFP, 2016a and 2016b). This includes both e-vouchers and cash transfers through financial service providers.
This article explores how the large quantities of data generated by transferring cash digitally could help transform humanitarian programme design, implementation and monitoring.
Both public and private sectors have used ‘big data’ analytics to great effect, for example, to detect fraud in the financial and insurance sectors. Big data has only seen limited use in the humanitarian sector however. To help change this, WFP and the Centre for Innovation (CFI) at Leiden University collaborated on researching how to support food security programming in Lebanon, using data derived from WFP’s cash-based transfer programme there.
The dataset: 6 million records from WFP’s e-card programme in Lebanon
Launched in 2012, WFP’s e-voucher programme in Lebanon is currently the agency’s largest cash-based transfer intervention; between US$18 and US$22 million is uploaded to e-cards every month.
As of early 2017, the programme was assisting 700,000 Syrian refugees, 54,000 Lebanese and 23,000 Palestinian refugees who can use e-cards to purchase food in some 500 shops located across the country. Each participating shop is equipped with at least one electronic payment device that clears transactions with the Banque Libano-Française.
Our study analysed the records of more than 6 million individual e-card transactions over an 18-month period from 2014 to 2015. Each transaction generated a record, which is geo-located, time-stamped and includes the monetary value of the transaction, as well as the reference of the device and shop where it was processed.
The raw dataset included some personally identifiable information so appropriate data protection precautions were taken. These included a non-disclosure agreement between WFP and CFI, removing personally identifiable information, ‘blurring’ locations by reducing the number of digits the GPS codes and secure data transfers.
Methodology: detecting anomalies in e-card transactions
Every WFP transfer programme faces instances of unintended behaviour. For example, beneficiaries or contractors sometimes take advantage of the programme by cashing out the credit provided. The Lebanon e-card programme is no exception. So, for our research, we decided to analyse data about monetary transactions between contracted shops and the bank, to determine whether it could be used to monitor retailers’ financial compliance with the programme.
WFP’s monitoring activities had already led to some retailers being disqualified for financial irregularities. We examined these shops’ transactions, to identify characteristics that could be used to identify others where financial irregularities could potentially occur. We noticed that many disqualified stores saw ‘high volatility’ in their sales, suggesting that large fluctuations in turnover could be a good indicator of unusual transactions.
In order to offer a ballpark estimate of the magnitude of the phenomenon, we estimated the total value of unusual transactions in the dataset. First, we identified high volatility shops. Secondly, in these shops, we calculated the sum of all ‘atypical’ transactions, those exceeding 50% of the median daily total of the shop where the purchase was made. As Figure 1 shows, high volatility shops had a total turnover of US$44 million over the 18-month period. The sum of atypical transactions was roughly US$11.2 million.
The number of atypical transactions is significant in programming terms; $11.2 million is equivalent to the cost of providing a US$27 transfer to 415,000 refugees for a month. However, US$11.2 million is a conservative figure; it only applies to stores identified as having highly volatile sales but atypical transactions could occur at many other stores that do not fall into this category.
However, it is important to note that atypical transactions are not necessarily instances of fraud, which require field verification to prove. In fact, there are legitimate reasons for sudden increases in a shop’s turnover, including a new influx of e-card users or the closing of a nearby shop.
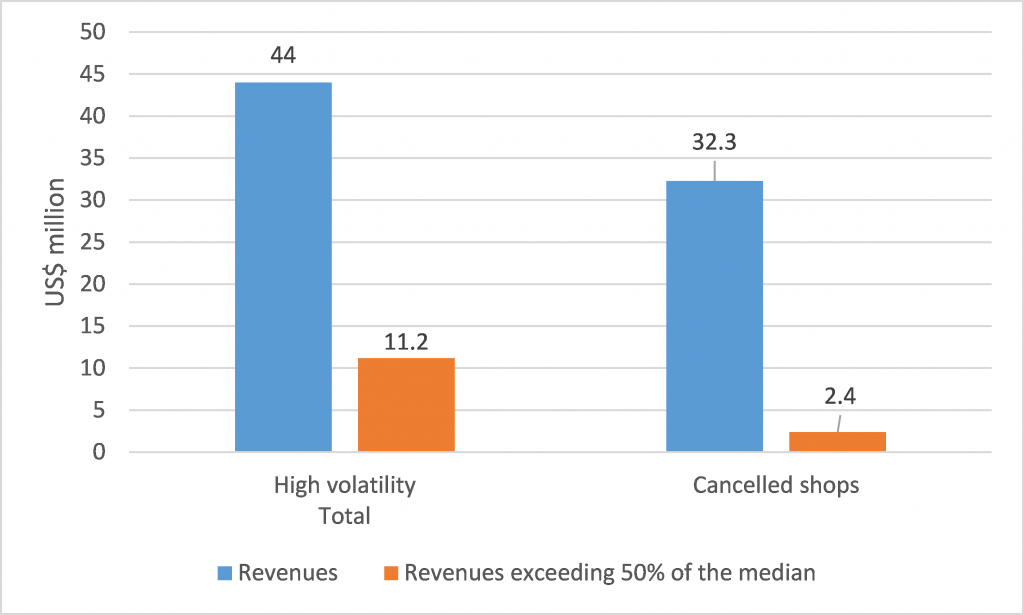
Towards real-time monitoring: automatically ‘flagging’ atypical transactions
Using these insights, WFP and C4I built a model that automatically identifies shops with atypical transactions patterns. Because the model can be run on transaction data that comes in from the bank, it is instantaneous, providing fresh information that helps direct in-person verification by field teams.
In specific terms, the model+ estimates the probability that a given shop’s daily revenue will exceed an expected value, with reference to the transaction date, the shop’s location, and size.
The analysis is run at the retailer level – rather than that of individual e-card holders. A sale at a shop is considered a random event. The model assumes that events are independent, that different people can spend their money at different participating shops of their choice at different times.
We used the model to flag shops with atypical transactions patterns. In the map below, shops flagged as outliers are highlighted in red, while those with normal transaction profiles are marked in green. Our analysis also suggests that the number of atypical transactions declined during the timeframe covered by the data because of better monitoring and tighter controls.
With frequent uploads of transaction data, the tool running the model now does so in nearly real time. It has been deployed as a prototype in Lebanon in September 2016.
The tool uses the above analysis of transaction patterns in addition to alerts set off by certain patterns, such as card swipes taking place late at night, or in rapid succession at a single point of sale. The system produces real-time reports that help field managers prioritise which retailers need closer monitoring.
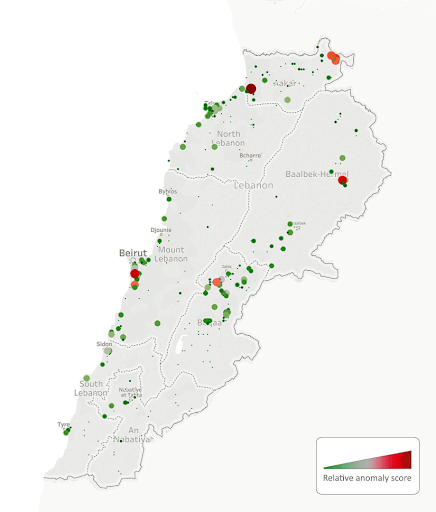
The tool also delivers insights into people’s movements within Lebanon. Large sets of e-cards that move ‘together’ from one shop to another over long distances could be a flag for atypical activity. However, there are also valid reasons for such activity, for example, a household might be relocating. Phoning the owners of e-cards is one way of checking whether there is cause for concern.
As well as flagging atypical transactions, this information could increase WFP’s understanding of how e-card users move around the country at different times of year. Figure 3 represents the dataset’s most common pairs of shops, i.e. every paired set of data points represents the two shops most regularly visited by e-card users from 2014 to 2015.
The figure reveals distinct ‘catchment areas’ around Beirut, Tripoli, Saida and the Bekaa Valley, as well as migration patterns within the country. For e-card users who have moved to a different part of the country, we can see lines traced between their old and new most frequented stores.
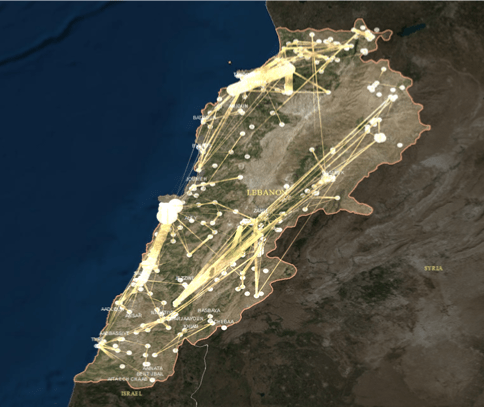
Why does this matter?
This article shows that, by following digital footprints, humanitarian agencies can monitor a programme’s performance and have atypical transactions automatically flagged – all in real time. This could improve programme accountability and efficiency, ensuring more resources reach those who need them most. Similar tools could be used to monitor unrestricted cash programmes; they might be used to analyse mobile money and ATM data, for example, as digital footprints exist in both cases.
In the United States, these techniques have helped reduce misuse of benefits provided by the Supplemental Nutrition Assistance Program (formerly known as food stamps) from 4% in 1993 to 1% in 2006-2008 (USDA, 2015). While humanitarian cash transfers are made in vastly different contexts, this example confirms that these technologies can cut down on fraud in digital transfer programmes.
Lebanon’s developed infrastructure, the size of WFP’s programme and existing human capacity make it an optimal place to test and refine these approaches, to help bring related platforms to scale in other humanitarian settings. Applying this form of monitoring elsewhere could also help WFP’s partners – including the private sector – monitor their programmes in an efficient and integrated way, while also contributing to the improvement of supply chains.
From programme compliance to empowering people
A major limitation of this system is that alerts are not proof of fraud. We are yet to establish the proportion of false positives generated, and further monitoring is always needed to validate the systems’ findings.
Looking to the future, integrating transaction data with other information – including food prices, household surveys and settlement data – could help contextualise findings. Collecting and analysing itemised receipt data could increase our understanding of people’s food preferences and inform assessments of seasonal variations in household consumption. Monitoring unrestricted cash transfers with these tools is also expected to be beneficial.
As humanitarian organisations increasingly use big data analytics, community outreach will become ever more important, so that the information that is collected also help people make better decisions. Should transactions analysis go into further detail – by including itemized food commodity receipts for instance – it is recommended to obtain explicit and informed consent from programme beneficiaries. More broadly, the application of big data analytics will continue to raise questions about the responsible management of personal information, especially for vulnerable groups, such as refugees.
A previous version of this article has been published on ODI-HPN.
Authors
Tobias Flaemig, Head, Economic and Market Analysis, WFP; Susanna Sandstrom, Economist, WFP; Oscar Maria Caccavale, Economist, WFP; Jean-Martin Bauer, Analyst, WFP; Arif Husain, Chief Economist, WFP; Arvid Halma, Data Analytics Lead, Center for Innovation, Leiden University; Jorn Poldermans, Project Manager, Center for Innovation, Leiden University.
References
- USDA (2015) http://www.fns.usda.gov/pressrelease/2011/050311
- WFP (2016a) Food assistance: cash based and in-kind https://www.wfp.org/cash-based-transfers
- WFP (2016b) WPF Mangement Plan 2016-2018 http://documents.wfp.org/stellent/groups/public/documents/eb/wfp277490.pdf