Projects
Using Social Network Analysis to Explore International Criminal Court Cases
In our work with the International Criminal Court, we piloted network modelling technology to capture the full picture of international legal cases. In this article, we report on Relext – a model that harnesses the power of social network mapping and language analysis technology.
International Criminal Court (ICC) cases are particularly complex and they deal with a huge number of documents, such as witness statements and NGO reports. Each of these elements are important factors in cases, as are the relationships between all of the actors involved. While making judgements, the ICC’s Judicial Divisions as well as other units of the Court need effective insights into the case. Traditionally, this is a highly labour intensive process, requiring large numbers of people to develop a full picture of the case.
To support this labour intensive process, we investigated the value of mapping the relations between actors. We applied social network analysis techniques on the documents containing information related to the case.
Challenges – variation and quantity
There are two major challenges in mapping the documents involved in cases brought to the ICC.
Variation – The documents span a variety of different formats. For instance, witness accounts, NGO reports, and news articles.
Quantity – The numbers of documents involved in these cases are very high.
What we developed
In order to analyse the information in the documents more efficiently, we developed Relext. We named it after an abbreviation of ‘relation extraction’ (a detection and classification technique).
Relext allows investigators to identify and extract the relations between actors, locations and actions from any kind of textual source. Highly detailed information from these accounts is extracted and captured as entities (nodes) and relationships (edges) into a graph database. Types of entities include, for example, persons, locations, organisations, events, and dates. By combining the data of the nodes and relationships it becomes possible to create a network overview of how entities relate to each other in a specific (criminal) event.
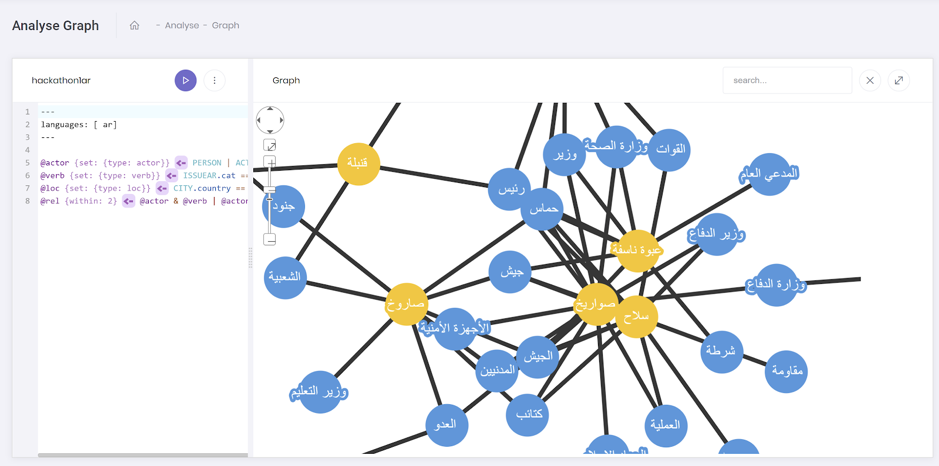
More than mapping?
What’s unique about Relextis that it uses social network analysis techniques in combination with Natural Language Processing (NLP) libraries (the same technology that powers chatbots). This makes it possible to extract items of interest as defined by the rules that the user sets up. For example, when an investigator is looking for the connection between military actors around a certain settlement, it can load the appropriate local dictionaries as set out by the rules and augment it by specifying names of interest. Machine learning models detect matching words to identify these text patterns at a large scale. This makes the analysis of a large number of data contained in different formats and languages far more efficient and much less labour intensive. Moreover, it makes the research more objective by formalising and classifying what the investigator is looking for.
Further application
Relext processes huge amounts of unstructured information to classified and quantitative information. Today many fields are challenged by an abundance of data, therefore the tool is highly relevant for many other domains as well. Thus, we see the Relext project as a starting point to explore other use-cases. Retrieving insights from customer reviews, for example, or moderating online community platforms by looking for a specific discourse.
Get in touch
Did this article interest you? Email us at c4i@sea.leidenuniv.nl or take a look at our team of experts.